EU3-Emilia Romagna region, Italy
The Civil Protection Agency of the Emilia Romagna Region recently implemented the SIGMA model in the ReLEW system, using a network of rain-gauges for the analysis of the amount of rainfall able to trigger landslides. The model is based on a set of statistical rainfall thresholds defined on the basis of a single parameter: the cumulate rainfall (Martelloni et al. 2012).
​
1 The setting
The Region was partitioned into 19 territorial units (TU), chiefly defined on the basis of different physiographic and environmental features (meteorological, lithological and geographical data) following the approach of previous works (Bertolini and Pellegrini 2001; Ibsen and Casagli 2004; Benedetti et al. 2005). However, TU boundaries always match with administrative borders (limits of municipalities). For each TU, an automated rain gauge was chosen to be used for the calibration of the model and, in the operative scenario, for the monitoring of the TU as well. Different criteria were considered to select the reference rain-gauge per each TU (Martelloni et al. 2012).
​
1 The modelling
The name of the model, SIGMA, reflects the central role of the standard deviations in the proposed methodology. The SIGMA model originates by the a.s.c.a.v method (Galliani et al., 2001), based on a statistical analysis of the cumulative rainfalls with an n-day wide moving window shifting at 1-day time steps along the whole rainfall record. Starting from the original series of daily precipitation (typically 1951–2009), the time series of cumulated data from 1 to 365 days was built for each TU reference rain gauge. The cumulative rainfall series are approximated to the standard Gaussian distribution through a target function (y = α∙σ), where α is a constant and σ is the standard deviation. From a particular value of σ or its multiples, the corresponding cumulative frequency sample is calculated and from this values the precipitation (in millimetres) of the original series. Proceeding in the same way for the number of cumulative rainfalls between 1 and 365 days, it is possible to build the precipitation curves (σ curves) associated with various probabilities of not being overcome (Fig. 13). Increasing values of the standard deviation (σ) are used as thresholds for issuing an alert level.

Figure 13: Example of rainfall probability curves (σ curves) in a cumulative period up to 100 days.
2 The warning strategy
The aforementioned σ curves are implemented in a decisional algorithm that constitutes the core of the SIGMA model. The latter operates separately for each TU, and in real-time applications, the model works at daily time steps providing a level of criticality that depends on weather forecasts and rainfall recordings. For each TU, these rainfall amounts are cumulated at increasing time intervals ranging from 1 to 245 days. Such cumulates are compared with the σ curves, which are actually used as thresholds. The decisional algorithm of the SIGMA model was developed to take into account both shallow and deep-seated landslides. In the decisional algorithm two different intervals of cumulative rainfall are considered: 1-3 days cumulates took into account the critical rainfall influencing shallow movements, whilst a variable time interval cumulate (up to 240 days) was used to consider the triggering of deep-seated landslides in low permeability terrains (Martelloni et al., 2012). For shallow landslides the equation (1) considers the cumulative daily rainfall precipitation of two days prior to the analysis.

For deep-seated landslides the algorithm takes into account the cumulative daily rainfall for a time interval varying with the season. During the dry season (May to October), the cumulative daily rainfall over a period of time ranging from 4 to 63 days is considered, while for the wet season, from the 1st November, the cumulative is increased by one per each day until a maximum (the 31st April) of 245 days (eq.2).

The algorithm provides a level of criticality on the basis of which σ curves are exceeded (if any), using the four alert levels adopted in the civil protection procedures: “absent”, “ordinary”, “moderate” and “high” (Fig. 14). The standard sigma curves considered by the algorithm (1.5, 2, 2.5, 3σ) delineate exceptional rainfalls with respect to the characteristics of each TU. The decisional algorithm is organized to provide increasing criticality levels with increasing rainfall amounts.
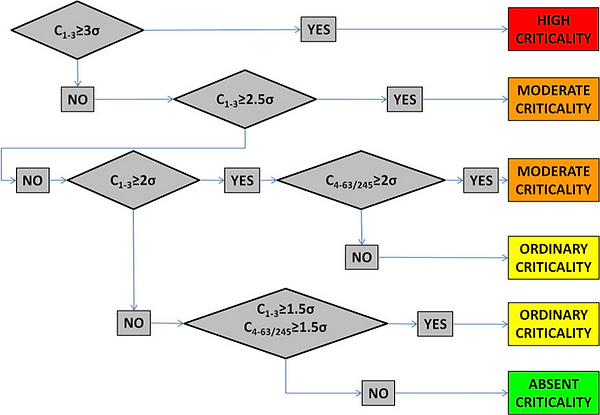
Figure 14: Decision algorithm of the SIGMA model. C1–3 indicates the rainfall cumulated from 1 to 3 days; C4–63/245 shows the rainfall cumulated from 4 to 63/245 days; 1.5σ, 2σ, 2.5σ and 3σ indicate the thresholds expressed in standard deviations
The correspondence between σ thresholds and expected effects to the ground (landslides) was better constrained performing a calibration with respect to dated landslides of the period 2004–2007. For each territorial unit, was compared the daily model outputs with the corresponding number of occurred landslides. At the first criticality level (ordinary criticality) the algorithm identifies the σ curves that minimize the occurrence of the threshold overcoming in days for which landslides were not reported, with a trial and error procedure, thresholds are progressively raised. Since no landslide was reported in correspondence with a rainfall event, the reference σ value was increased until the cumulative rainfall curve did not overcome the threshold. The procedure is repeated for every event generating a false alarm with the constraint of not reducing the number of the correctly detected landslides. It is important to highlight that the calibration procedure does not change the sigma curves, but it chooses a customized set of sigma curves for each TU.
The model was validated using landslides and rainfall recordings from the period 2008–2010. In the validation test, the SIGMA model was run with the rainfall recorded by the reference rain gauges in the period 2008–2010, and for each TU, the daily alert level provided by the decisional algorithm was compared with dated and geo-registered landslides from the same period, which were organized in a constantly updated geo database. Correct predictions (true positives and true negatives) and errors (missed alarms or false negatives and false alarms or false positives) were consequently defined and summarized in terms of number of landslides and number of days (in a single TU each day has an alert level but more than a single landslide can happen). In the validation period 764 landslides occurred, and 84% of them were correctly predicted. The examination of the results of the model validation revealed a satisfactory correspondence between days with landslides and alert levels provided by the decision-making algorithm. In addition, the comparison between the validation results and the Monte Carlo simulation reveals that the criticality levels provided by the SIGMA model are far from a random distribution (Martelloni et al., 2012).
​
3 The response strategy
For each reference rain gauge, software combines rainfall recordings from the regional automated network with rainfall forecasts and compares the resulting cumulative rainfalls with the thresholds. In the territorial units where the latter are exceeded, the software provides the corresponding alert level, according to the decisional algorithm (Fig. 14), and then the Regional Civil Protection Headquarters weigh up these SIGMA outputs. Normally, at the ordinary criticality level no particular countermeasure is undertaken except for a more frequent monitoring activity, while moderate and high criticalities can be converted in real alerts addressed to municipalities and to other environmental agencies.
​
References
Benedetti A, Casagli N, Bosi V, Dapporto S, Ciolli S, Palmieri M, Zinoni F (2005) Modello statistico per la previsione operativa dei fenomeni franosi nella regione Emilia-Romagna. Boll Soc Geol Italy 124:333–344
Bertolini G, Pellegrini M (2001) The landslides of the Emilia Apennines (Northern Italy) with reference to those which resumed activity in the 1994–1999 period and required civil protection interventions. Quaderni di Geologia Applicata 8(1):27–74
Galliani G, Pomi L, Zinoni F, Casagli N (2001) Analisi meteoclimatologica e soglie di innesco delle frane nella regione Emilia-Romagna negli anni 1994–1996. Quaderni di Geologia Applicata 8:75–91
Ibsen M-L, Casagli N (2004) Rainfall patterns and related landslide incidence in the Porretta-Vergato region, Italy. Landslides 1:143–150
Lagomarsino, Segoni, Fanti, Catani (2013) Updating and tuning a regional scale landslide early warning system. Landslides. 10, 91–97. DOI: 10.1007/s10346-012-0376-y.
Martelloni, Segoni, Fanti, Catani (2012) Rainfall thresholds for the forecasting of landslide occurrence at regional scale. Landslides 9(4), 485-495. DOI: 10.1007/s10346-011-0308-2